Hammers & Nails 2023 - Swiss Edition
Congressi Stefano Franscini (CSF)
Frontiers in Machine Learning in Cosmology, Astro & Particle Physics
October 29 – November 3, 2023 | Conference center Congressi Stefano Franscini (CSF) in Monte Verità, Ascona, Switzerland
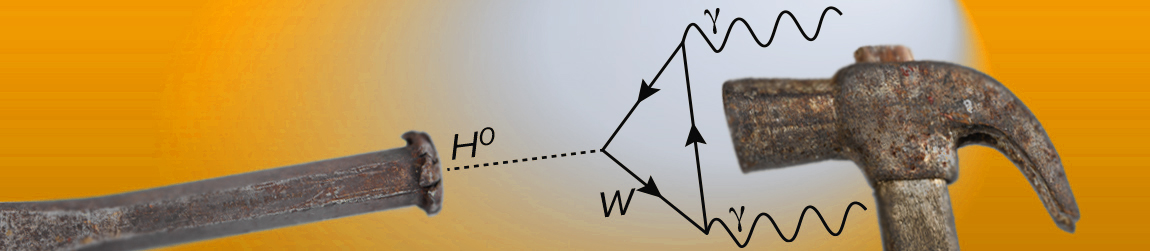
The Swiss Edition of Hammers & Nails in 2023 is following the success of the 2017, 2019 and 2022 Hammers & Nails workshops at Weizmann Institute of Science, Israel.
Cosmology, astro, and particle physics are constantly pushing forward the boundary of human knowledge further into the previously Unknown, fueled by open mysteries such as dark matter, dark energy or quantum gravity: simulating the Universe, simulating trillions of LHC particle collisions, searching for feeble anomalous signals in a deluge of data or inferring the underlying theory of nature by use of data which has been convolved with complex detector responses. The machine learning hammer has already proven itself useful to decipher our conversation with the Unknown.
What is holding us back and where is cutting-edge machine learning expected to triumph over conventional methods? This workshop will be an essential moment to explore open questions, foster new collaborations and shape the direction of ML design and application in these domains.
An overarching theme is given by unsupervised and generative models which have excelled recently given the success of transformers, diffusion and foundational models. Other success stories include simulation-based inference, optimal transport, active learning and anomaly detection. The community has also taken inspiration from the rise of machine learning in many other domains such as in molecular dynamics.
The trademark of Hammers & Nails is an informal atmosphere with open-ended lectures spanning academia and industry, and a stage for early-career scientists, with time for free discussion and collaboration.
Participation is by invitation. Limited admission through submission of an abstract and a brainstorming idea is available with focus on early-career scientists.
Confirmed invited speakers and panelists:
- Thea Aarrestad (ETH Zürich)
- Piotr Bojanowski (Meta AI)
- Michael Bronstein (University of Oxford | Twitter)
- Taco Cohen (Qualcomm AI Research)
- Kyle Cranmer (University of Wisconsin-Madison)
- Michael Elad (Technion)
- Eilam Gross (Weizmann Institute of Science)
- Loukas Gouskos (CERN)
- Lukas Heinrich (Technical University of Munich)
- Shirley Ho (Center for Computational Astrophysics at Flatiron Institute)
- Michael Kagan (SLAC)
- Francois Lanusse (CNRS)
- Ann Lee (Carnegie Mellon University)
- Laurence Levasseur (University of Montréal | Mila)
- Qianxiao Li (National University of Singapore)
- Jakob Macke (Tübingen University)
- Alexander G. D. G. Matthews (Google Deep Mind)
- Jennifer Ngadiuba (Fermilab)
- Kostya Novoselov (University of Singapore | Nobel laureate
- Mariel Pettee (Berkeley Lab)
- Barnabas Poczos (Carnegie Mellon University)
- Jesse Thaler (MIT)
- Andrey Ustyuzhanin (Higher School of Economics)
- Ramon Winterhalder (UC Louvain)
Scientific Organizing Committee:
- Tobias Golling (University of Geneva)
- Danilo Rezende (Google Deep Mind)
- Robert Feldmann (University of Zurich)
- Slava Voloshynovskiy (University of Geneva)
- Eilam Gross (Weizmann Institute of Science)
- Kyle Cranmer (University of Wisconsin-Madison)
- Ann Lee (Carnegie Mellon University)
- Maurizio Perini (CERN)
- Shirley Ho (Center for Computational Astrophysics at Flatiron Institute)
- Tilman Plehn (University of Heidelberg)
- Elena Gavagnin (Zurich University of Applied Sciences)
- Peter Battaglia (Google Deep Mind)
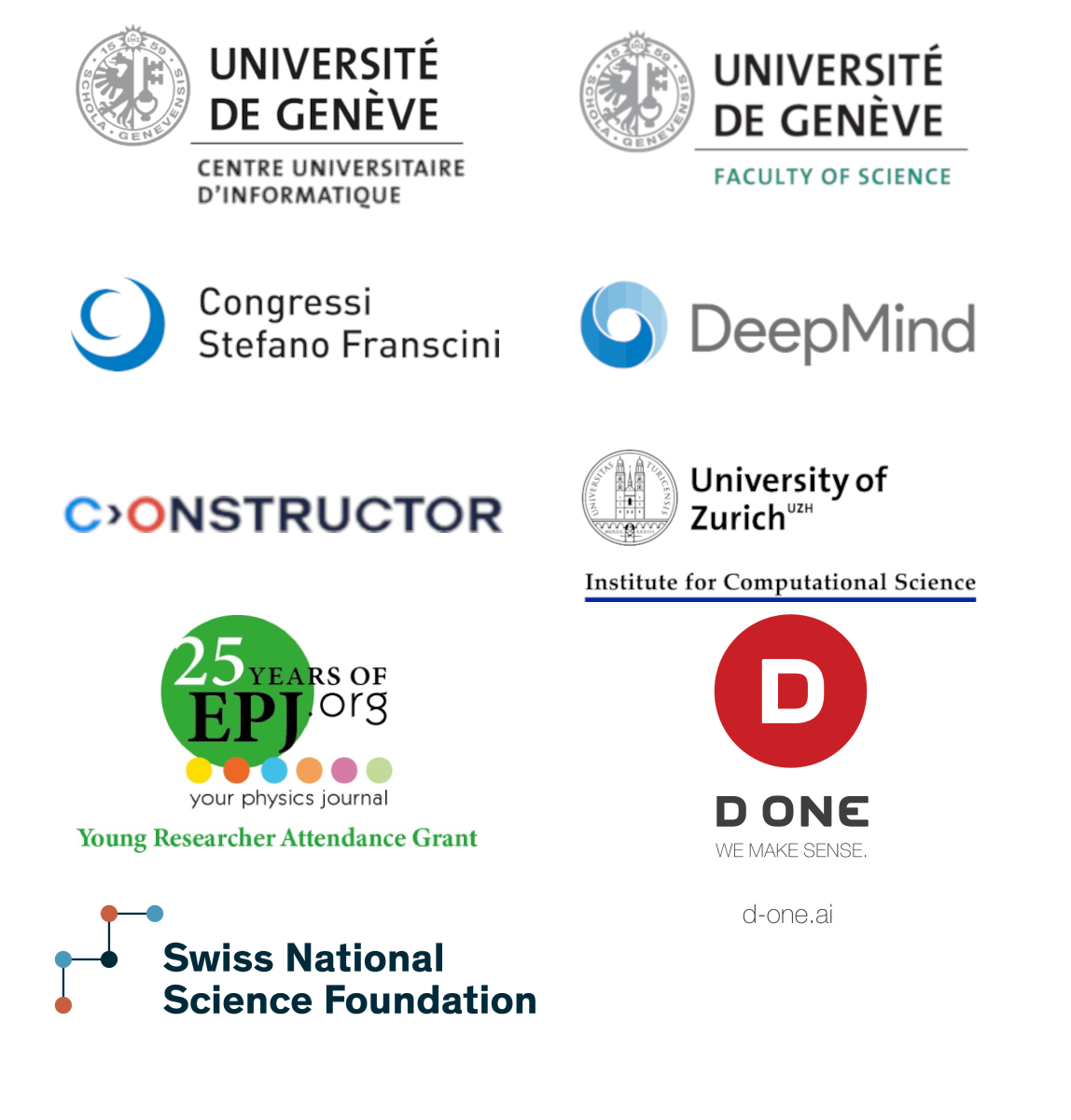